Projects
The Neural Basis of Semantic Representation
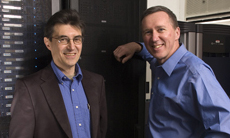
Our approach applies machine learning algorithms to fMRI data to identify neural patterns associated with individual concepts. The computer program learns a representation from a subset of our fMRI data and can then identify a novel brain image it has not yet seen. We have also developed a computational model that predicts fMRI activation of words for which fMRI data are not yet available (Mitchell et al., 2008). By leaving out different subsets of images to test the program, we obtain a measure of how well our algorithms can generalize to new data, or an independent subset of the same participant's data (Just et al., 2010). The predictions consistently achieve a high level of accuracy, and generalize in interesting ways: we can obtain successful predictions when training on a different language in bilingual participants, when training on one modality to predict another (words and pictures), and training on one study to predict another study with different stimuli.
Brain imaging and machine learning thought reading video.
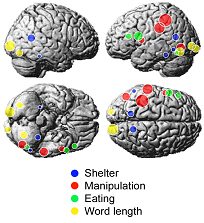
The continued success across these domains indicates that we have uncovered a robust neural representation of several dimensions of the semantic space. Current work includes mapping along other dimensions, investigating how individual words combine into sentences, and integrating these findings into computational models of language comprehension.